Hamda's paper evaluating genetic interaction scoring systems for combinatorial CRISPR screens
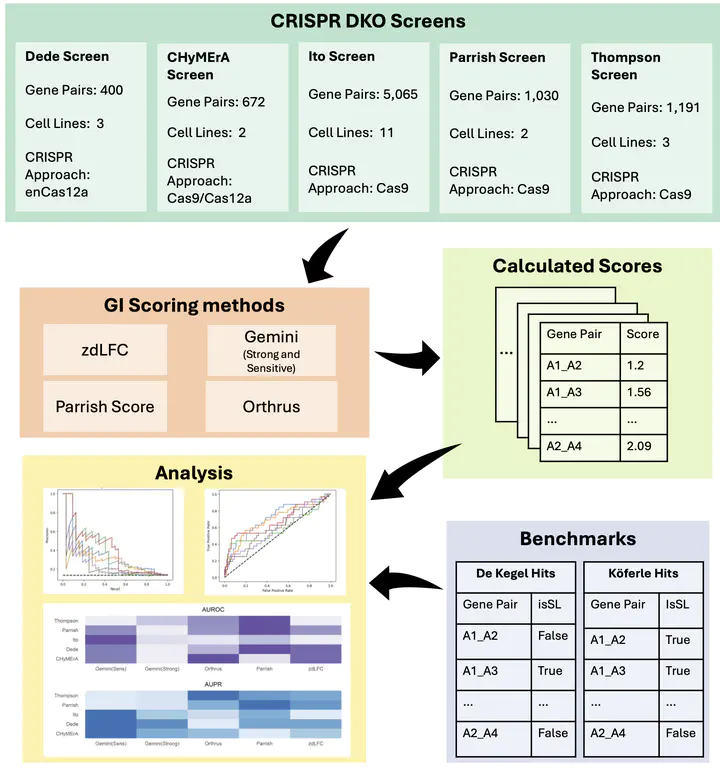
Congrats to Hamda on her first publication with the lab, Benchmarking Genetic Interaction Scoring Methods for Identifying Synthetic Lethality from Combinatorial CRISPR Screens, up now on biorXiv.
Combinatorial CRISPR screens, where two genes are perturbed simultaneously, are increasingly used to identify new synthetic lethal targets in cancer. They’ve been particularly popular for identifying synthetic lethal relationships between paralog pairs. In order to identify synthetic lethal hits from these screens it is necessary to identify and quantify genetic interactions – instances where the effect observed from perturbing two genes diverges from what is expected based on perturbing each gene individually. Multiple scoring pipelines for quantifying genetic interactions from these screens have been published, but comparison across pipelines has been limited (in part due to the lack of an external benchmark). Indeed most published screens use distinct scoring systems, making their results difficult to compare.
In this work we propose that paralog synthetic lethal interactions identified from DepMap single-gene CRISPR screens can be used as an external benchmark. These pairs have been found to be synthetic lethal across cell lines from diverse cancer types by associating the loss of one gene with increased sensitivity to inhibition of another. They are therefore at least somewhat robust to genetic background and unlikely to be cell line specific. This is not a perfect benchmark, as some pairs that are synthetic lethal in only specific contexts will be missed, but overall gene pairs found to be synthetic in a panel of cell lines should get a higher score than those where there is no evidence of synthetic lethality in the panel.
To compare scoring systems using this approach, Hamda rescored 5 published combinatorial CRISPR datasets (total 21 screens) using 5 distinct scoring systems. Getting the scoring systems to work across multiple screens and rescoring everything was a lot of work but allows us to compare scoring systems in a systematic fashion. We found that no single method performs best across all screens but identify two methods that perform well across most datasets.
You can read the full paper here for all of the comparisons!